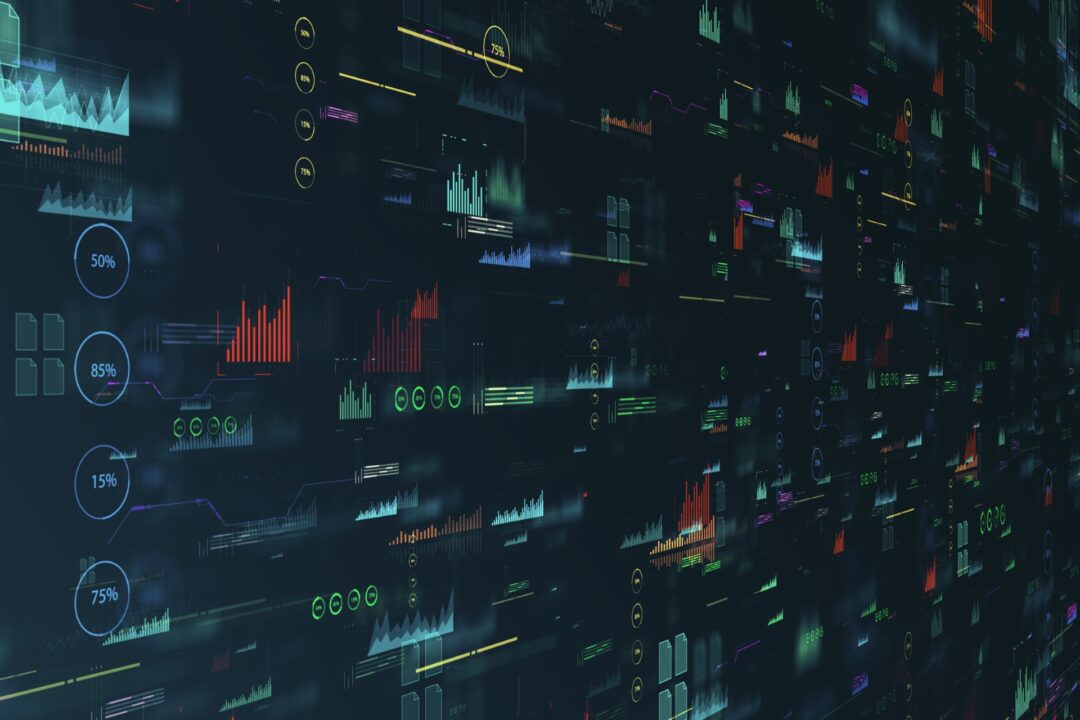
Managing mountains of data
In the second article of our data assurance series covering law enforcement data challenges, we discuss how to use technology to manage large amounts of data that enable investigators to focus their efforts on getting results.
The data deluge
Data volume is increasing every day and is being generated by more sources than ever. Formats now include IoT and machine data, social media feeds, CCTV footage, seized media from phones, laptops, and cars. Demand from law enforcement is such that data collected must be fused together and translated straight away. If you’re exhausted just thinking about it, imagine the analysts who live in this reality.
Luckily there are methods we can use to work through this challenge. Automation of tasks is already underway in a number of industries to speed up process stages.
We can apply similar techniques to the data lifecycle, from automating the initial gathering stage to setting up continuously-updating real-time dashboards to provide up-to-date insight. The trickiest and most valuable stage lies in finding the needle in the haystack.
We have developed tools such as STARTLE® and Media MinerTM that embed into human teams with the goal of reducing the haystack to a manageable amount, allowing humans to focus where they’re needed most. This is often referred to as Human-Machine Teaming.
A balancing act
In these cases, we need to balance speed, accuracy, and thoroughness. With thoroughness comes greater accuracy, but you don’t necessarily need to be thorough to be accurate enough. This is where the balance comes in. Generally, greater accuracy and thoroughness also come at the cost of speed. You could also take the ‘Big Data’ approach and distribute your processing, but this still requires investment in compute resource.
There are some key questions to answer here in order to understand the required approach.
- Do you need to have looked at all of the data?
- What is your threshold of accuracy for machine processes?
- What is your false positive/false negative ratio?
In order to determine how much data you need to process, it’s key to consider your domain. In the world of law enforcement, it’s crucial to understand both what is and isn’t important to your investigation. If something has been overlooked which later turns out to be important, it can throw the entire investigation into question.
In opposition to this, it’s crucial to gain insight so timely action can be taken and without exhausting human operators. Machines can run continuously, overnight, and don’t need breaks – this makes them ideal for heavy processing tasks that don’t require human input. Tools like Media MinerTM process data at scale in order to provide directions to human operators so that they can home in on key evidence and intelligence quickly.
Accuracy requirements are similarly influenced by the scenario. For example, if you were working with social media accounts trying to determine whether one was a bot, a false positive would be an account labelled as a bot when it isn’t, and a false negative would be an account being labelled as ‘not a bot’ when it is. Your situation will often dictate which of these is of higher risk which can determine the appropriate approach. Often this is a hybrid situation where humans and machines work together to solve an issue. In cases where it’s far more important not to miss something, you may want to have the machine bring back more results (with the aim of reducing your false negatives, though likely increasing your false positives) which the human can then verify. The results from this process can then be fed back to the machine to improve future findings.
Providing trust and reassurance
As we move to blended teams of humans and machine intelligence, we must look to provide trust and reassurance in the algorithms we apply. Methods for assuring the user may vary from overlaying heatmaps (to demonstrate areas of interest) to natural-language step-by-step explanations as to why a decision was reached (often dictated by the type of data). This allows the human operator to better understand why something has been highlighted to them, but also gives scientists and developers a crucial insight on future improvements.
STARTLE® provides a threat warning along with the reasoning behind it, showing both awareness and understanding to human operators. This explanation saves crucial time for decision makers and brings machine processes to a level of trust where they can act as members of the team. We follow an approach of Explain, Assure, and Protect when it comes to developing AI services so users can trust the result.
It’s important to understand the legal constraints when making use of automation, particularly in law enforcement. Where data is used in evidence, the investigator must be content to stand up in court and explain the evidence in detail.
By producing a workflow alongside the algorithm the investigator gains an understanding of what the algorithm is doing at every stage in its process.
In intelligence operations we must also take care to avoid bias and be conscious of privacy regulations. Legal frameworks such as the Equality Act and GDPR can quite easily be overlooked when developing and deploying automation technology, as evidenced recently with South Wales Police’s use of Automatic Facial Recognition. This is why it is important to undertake both the Explain and Assure steps in your approach: can you explain what your algorithm is doing, and can you be sure it’s free from bias?
Technology has advanced rapidly to meet the demands of modern data and continues to evolve. A key area of our research is ensuring those technologies and techniques remain a viable solution for law enforcement. Adoption of automation and exploitation is key to future investigations as data challenges continue to advance, but the challenge of building in trust must be addressed.
Conclusion
Roke’s data assurance automation solutions—featuring STARTLE® and Media Miner™—enable law enforcement to rapidly process vast multi‑source data, extract actionable intelligence, and maintain courtroom-grade evidence integrity through explainable AI, boosting investigator efficiency, operational trust, and mission impact.
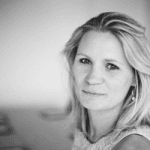
Omi Ducat
Chief Marketing Officer