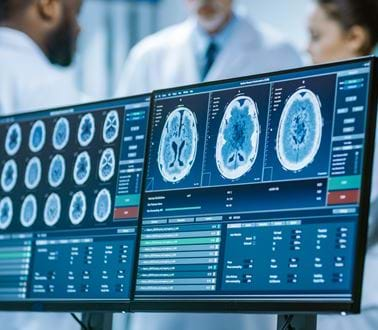
The Challenge
In a 2019/20 report1, NHS Resolution reported that the value of payments (damages, claimant, and NHS legal costs) were £2.3 billion for clinical negligence and £52m for non-clinical negligence. In addition, the average time lag for clinical cases was 2.7 years, rising to 6.3 for brain injuries to babies, or 11 years for Erb’s palsy, meaning that serious harm in a cluster may not be picked up for many years. ‘Intelligence’ was highlighted as a key strategic priority to provide analysis and expert knowledge to drive improvement in these areas. To support this theme, Roke was assigned to a project focussing on predicting the level of clinical claims based on available NHS data sets.
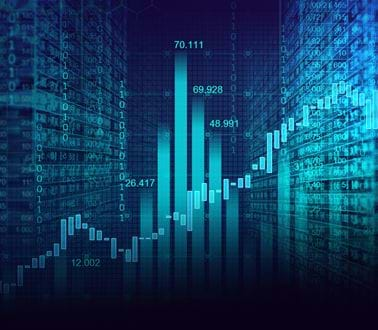
The Approach
Over an eight week period, our team developed the capability to upload multiple data sources and rapidly generate automated machine-learned models to classify the data. This was a key element to our way of working as it provided immediate insight while simultaneously preparing other data sources for inclusion. Using automated machine learning, and working against a single data set meant we could continuously deliver new capability. We also incorporated our agile approach, working in sprints, demonstrating and discussing the latest increments and findings to the customer each week. Over 20 sources of data were made available to the team which we steadily consumed in priority order to prepare them for ingestion by the machine-learned model.
Crucially, the team also provided automated insight to explain the classification output of the machine-learnt models including details on how certain fields influenced the classification. This proved useful and effective for understanding the outcomes.
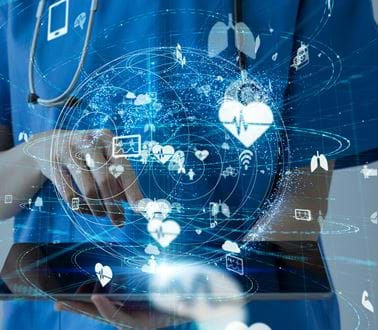
THE OUTCOME
Our approach meant that we were able to provide repeatable capability for processing and ingesting multiple data sources on negligence claims. This capability can now be used by a machine-learnt model to provide insight on the likelihood of a particular NHS Trust being at high risk of a large number of claims.
In the short timeframe, we also identified a set of key features that could suggest areas where those Trusts could be at a higher risk, providing business intelligence to inform decision makers on possible improvements. NHS Resolution is able to reuse this code base and methodology with future datasets with a view to providing intelligence to the wider community and making decisions to improve existing services.
By fusing these datasets across multiple sources and developing a machine-learned algorithm for interpreting this information, we hope that it will aid NHS Resolution in the future. This project was a starting point in better understanding the picture presented by collated data and the value it may give going forward in relation to repeatable insight informing decisions that can improve NHS services.
Giuseppe Sollazzo, Head of Skunkworks, NHS AI Lab, NHSX
“Roke's report was especially useful as it highlighted not just that the data could broadly be used for better-than-baseline forecasts, but it also suggested a number of actionable ideas on how to improve data quality, something we really care about because without good data there cannot be good AI."
Related news, insights and innovations
Find out more about our cutting-edge expertise.
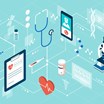